xgnlab
Global Community of People and Entities Contributing for Next Generation Networks Ecosystems - 4G/5G. Any Member, individual or a company, here has no liability to others and even to xgnlab, vice a versa, it's a platform to showcase or share their work and knowledge only.
GA
Translate
Recent Most Popular
-
Operational Imperatives for 5G - A Report from TM Forum
-
BRUSSELS – October 23, 2018 – At its recent General Assembly, 5G IA elected its new Board for a term of two years until October 2020. 5G...
-
FCC chairman Mr Pai, known for his quick and fast decision making capabilities and abilities, said here in INDIA IMC 2018, that he does not ...
-
Akraino Edge Stack , a Linux Foundation project initiated by AT&T and Intel, intends to develop a fully integrated edge infrastructur...
-
WBA has organized the City Wi-Fi Roaming trial under the World Wi-Fi Day initiatives across many cities since 2016. The City Wi-Fi Roamin...
-
Vodafone is rolling out Ericsson's new compact antenna to bring greater 5G capacity, coverage and performance to locations across the ...
-
Hypervisors are a way to manage virtual machines (VMs) on processors that support the virtual replication of hardware. Not all processo...
-
In this article, we address a critical gap within the existing literature by offering a dedicated treatment of the 3GPP Release-18 study o...
-
White paper vindictively stress on convergence for 5G step wise growth and strategical adoption. Convergence, not only aggregation at acce...
Monday 14 August 2023
An Overview of the 3GPP Study on Artificial Intelligence for 5G New Radio
Saturday 12 August 2023
Ericsson Green initiative for 5G roll outs, helping Vodafone UK.
Vodafone is rolling out Ericsson's new compact antenna to bring greater 5G capacity, coverage and performance to locations across the U.K. The Ericsson AIR 3218 combines a radio unit and antenna in a single unit. It can also transmit mobile data over all of the frequencies that Vodafone currently uses in the U.K., without needing additional antenna units, as was the case for previous models.
The combined multiband, Massive MIMO design makes it easier for the operator to add more capacity to a mast without increasing its footprint. It's also easier to mount on rooftops, towers, walls and poles.
"5G is the UK's digital future, but we should never underestimate how difficult it is to deliver a future-proofed network at scale across the length and breadth of the UK. Working in partnership with Ericsson, we are constantly exploring new ways to accelerate this transformation, and this is another example of where innovation is delivered through collaboration," said Ker Anderson, head of Radio and Performance at Vodafone UK, in a statement.
Wednesday 8 February 2023
What is Milimeter Wave Bands for Next Gen ( 5G and beyond) Wireless Systems
Fifth Generation (5G) cellular systems are being designed to communicate over both sub-6 GHz bands (a.k.a. Frequency Range 1) as well as mmWave bands (a.k.a. Frequency Range 2). mmWave spectrum offers abundant bandwidth, which can be used to support multi-Gbps transmission speeds per user. Historically, mmWave bands have been used for fixed/mobile satellite services (FSS/MSS) and local multipoint distribution service (LMDS). Recently, the Federal Communications Commission (FCC) has opened up nearly 11 GHz of the mmWave spectrum for mobile broadband, aiming to support 5G cellular systems, wireless LANs (e.g., Wi-Gig), and others. The newly allocated bands include licensed bands at 24 GHz (24.25-24.45 GHz and 24.75-25.25 GHz), 28 GHz (27.5-28.35 GHz), 37 GHz (37-38.6 GHz), and 39 GHz (38.6-40 GHz), as well as a new unlicensed band at 64-71 GHz – see Figure 1. Combined with the previously introduced 57-64 GHz unlicensed band, this creates almost 18 GHz of new spectrum for next-generation wireless systems. The FCC is also planning to add 15.8 GHz more spectrum at 31.8-33.4 GHz, 42-42.5 GHz, 47.2-50.2 GHz, 71-76 GHz, and 81-86 GHz bands, along with spectrum above 95 GHz.
Figure 1: Licensed and unlicensed bands in the mmWave spectrum.
Despite this abundant capacity, RF communications at mmWave frequencies can be quite challenging, due to high propagation losses, poor penetration, blockage, rain sensitivity, etc. At the same time, the smaller wavelengths make it possible to pack tens or even hundreds of antenna elements into a single device (mobile phone, Small base station, or access point). With proper processing of signals fed into these antennas, electronically steerable, highly directional transmissions can be performed. The severe signal attenuation can, thus, be compensated for by the resulting beamforming and spatial reuse gains.
There are several ways to apply beamforming at mmWave frequencies. Fully digital beamforming relies on the availability of several RF chains. A particular precoding vector is multiplied by the modulated baseband signal of a given RF chain. Analog beamforming, on the other hand, can be performed with a single RF chain. It applies the beamforming weights in the RF domain by controlling phase shifters. Finally, in hybrid (analog/digital) beamforming, the signal processing is divided between the analog and digital domains, allowing comparable performance to digital beamforming but with fewer RF chains. The low power consumption of the analog beamforming makes it a desirable architecture, especially for the user equipment (UE).
What is CD-SSB in 5G NR?
Wednesday 25 January 2023
verizon pvt 5G strategy for industry 4.0
Thursday 22 December 2022
CSI-RS Classification and Role - 5G NR
Wednesday 21 December 2022
5G- Beam forming, Beam Steering, and MIMO - simplified.
Saturday 27 June 2020
WBA OpenRoaming - An ubiquitous WiFi experience across the world
What is OpenRoaming?
- Higher quality of service
- No need to track or switch among SSIDs
- A single log-on experience
- Business incentives, primarily through simplicity, to adopters
- Ease of regulatory compliance
- Passive authentication
Tuesday 12 March 2019
5G Race, from marketing stunt to political mileage.
Almost since 5G first grabbed the industry's attention, US telcos have been crowing about their investment in the next-generation mobile standard. And, rightly or wrongly, an impression has taken hold that America is a frontrunner in the 5G race. Only this week, a report from Arthur D. Little, a respected consultancy, ranked the US just behind South Korea in its new 5G country leadership index (see below). Other commentators point to major equipment deals for Ericsson and Nokia, and enthusiastic announcements about 5G launches, as evidence of the US position.
But it's all just marketing flimflam, according to one of the UK's top executives involved in the rollout of 5G technology. Light Reading spoke with Scott Petty, the chief technology officer of Vodafone UK, on the sidelines of a press briefing in London this week, and his verdict on claims of US 5G leadership was damning. "Only the Chinese are ahead of the UK. The US is miles behind," Petty told Light Reading. "They are making it up. They are rebadging 4G Evolution as 5G."
This would seem like chest-thumping for the UK telecom sector were it not for the fact that US boasts have never quite made sense.
For one thing, the 5G equipment vendor that just about every non-US telecom executive thinks of as the world's best -- China's Huawei -- is effectively barred from doing business with the main US operators. Elsewhere, even telcos ripping out Huawei's equipment, or promising not to use its 5G products, rank it ahead of Ericsson and Nokia. How can a country that excludes the world's "only true 5G vendor," in the words of BT's Neil McRae, be a 5G leader?
Very nicely put, get full article HERE
Monday 11 March 2019
Akraino Edge Stack from Linux Edge Linux fundation
- Telco NFV Edge Infrastructure - Running cloud infrastructure at the network edge allows for the virtualization of applications key to running 5G mobility networks at a larger scale, density and lower cost using commodity hardware. In addition this infrastructure can also enable the virtualization of wireline services, Enterprise IP services and even supports the virtualization of client premises equipment. This reduces the time to provision new services for customers and even, in some cases, allows those customers to self-provision their service changes.
- Autonomous devices - Drones, Autonomous Vehicles, Industry Robots and such customer devices require a lot of compute processing power in order to support video processing, analytics and etc., Edge computing enables above-said devices to offload the computing processing to the Edge within the needed latency limit.
- Immersive Experiences - Devices like Virtual Reality (VR) headsets and Augmented Reality applications on user’s mobile devices also require extremely low levels of latency to prevent lag that would degrade their user experience. To ensure this experience is optimal, placing computing resources close to the end user to ensure the lowest latencies to and from their devices is critical.
- IoT & Analytics - Emerging technologies in the Internet of Things (IoT) demands lower latencies and accelerated processing at the edge.
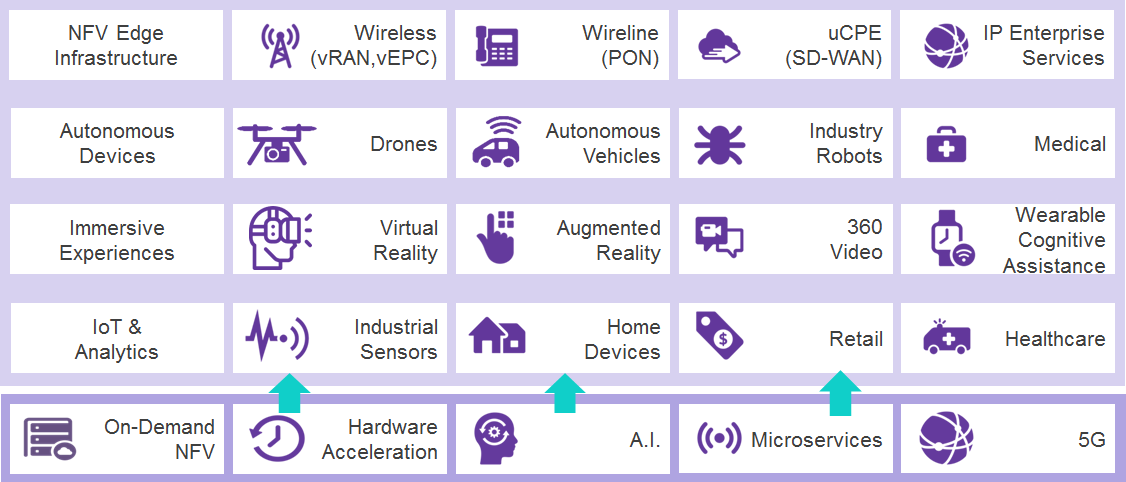
Network Edge - Optimal Zone for Edge Placement
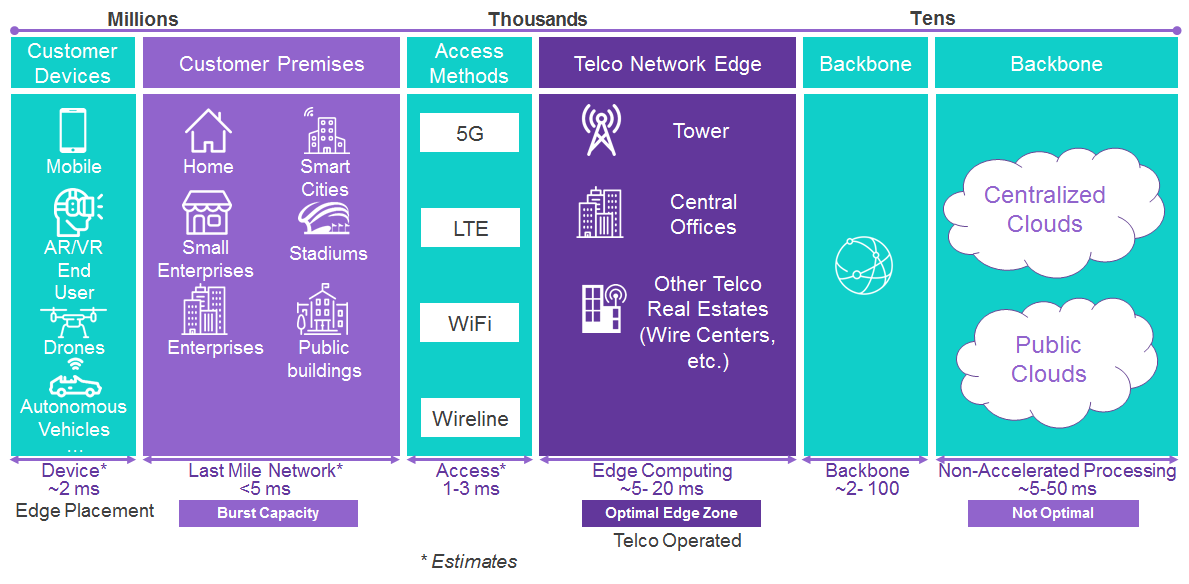
Akraino Edge Stack
